Starlink D2C, Tower Si-Pho, LLMs for Chip Design, Ionosphere Maps with Cellphones, Symmetry in IC Design
FCC gives Starlink a green light; 300 mm silicon photonics from Tower; AI driven chip design; using cellphones as earth sensors; tools to ensure symmetry in analog design.
Happy Thanksgiving to my US readers! We have crossed 4000 subscribers to this newsletter, and I thank you all for your readership and support.
This week’s post is different from the usual tech explainers I write. It is a collection of news and interesting articles I came across during my internet travels and article research. A lot of what I read during any given week does not make it into the weekend edition of the newsletter because it does not fit the narrative, is tangential to the topic, or even completely unrelated. The internet is rife with so many interesting things that I figured it will be useful if it is compiled into something you can easily skim through.
If you think I should continue doing posts like these, please leave a comment, or reply to this email and let me know!
Here’s a quick list of today’s topics:
Starlink gets FCC approval for commercial D2C
Tower commercializes 300 mm silicon photonics platform
Will LLMs for chip design actually work?
Mapping the ionosphere with millions of android phones
Tools to check symmetry in analog and RF IC design
Read time: 9 mins
Starlink gets FCC Approval for Commercial D2C
There has been a race to set up cell towers in space and provide internet access to underserved areas in the world, especially in areas where there is insufficient telecom infrastructure. This technology is called direct-to-cell (D2C) and it can also be used to bolster signal reception in weak spots by providing supplemental cellular service (SCS).
Ben Longmier, Senior Director of Satellite Engineering at SpaceX recently posted on X announcing that FCC has granted a commercial license to Starlink for D2C services.
This announcement comes in the light of the ongoing debate about Starlink’s request to the FCC to waive the out-of-band emission (OOBE) spec to prevent interference to existing terrestrial networks. Specifically, Starlink is proposing an emission spec that is almost nine times higher than the current levels stating that the FCC spec is not rooted in physics, and that tests on T-mobile networks show no noticeable degradation in terrestrial mobile service. This wavier request has been opposed by several American and European satellite and telecom providers who say that quality of existing service will drop significantly. I wrote in detail about both sides of the argument below.
The FCC filing states that the decision to allow or disallow increased OOBE levels has been deferred. This means that Starlink D2C will have to operate within the current OOBE power flux density specification set by the FCC at -120 dBW/m2/MHz. To meet this spec, Starlink has said they need to lower their transmit power levels which will limit D2C to text-only services. We will need to wait and see what this technology is actually capable of when it gets into the beta testing phase.
Tower Commercializes 300 mm Silicon Photonics Platform
Silicon photonics is seeing rapid growth due to rising needs for data input and output in datacenters and AI hyperscalars. There is a need to quickly and efficiently transport data between chips, modules, server racks or even between datacenters. Optical technology has been the backbone of data communication networks a long time, but the future holds a lot more photon based data transport especially considering the need to rein in datacenter energy costs.
Tower Semiconductor has announced a 300 mm photonics process as a commercially available foundry node to meet the needs of high speed data communications. It essentially uses their SiGe process flow for integrating photodetectors, modulators and lasers into a single die. Arguably, Tower is still playing catch-up with GlobalFoundries’ 300 mm Fotonix platform that was announced over two years ago, and has already seen adoption by “hot” startups such as Lightmatter who have partnered with GlobalFoundries for their photonics interconnect platform.
Still, it is encouraging to see more major fabs provide options for lower cost optics and photonics applications that clearly seem to be in demand. Meanwhile, if you’re new to photonics and want to watch a great introduction to the topic, check out the video below.
Will LLMs for Chip Design Work?
Y-combinator has highlighted “LLMs for chip design” as one of the many cool ideas that is possible in the Golden Age of Building. Coincidentally there has been a rise in startups developing chip design tools, when in the past, no one dared enter the space dominated by Cadence and Synopsys. Even Cadence is focusing on utilizing AI to accelerating chip design, and is likely that if any of these startups succeed in developing tools that make a meaningful improvement to chip design productivity, they’ll probably get acquired by the big two EDA companies.
However, progress on generative EDA using AI has been slow, the primary cause being the lack of adequate training data. Companies doing chip design closely guard their design IP and this makes it difficult to train a LLM to perform digital design, verification and RTL synthesis at the levels humans do. In the world of analog, RF and photonics, this is even harder to do because of tacit knowledge involved in human design. The return on investment is even questionable in the analog world considering how much more niche it is, in the context of the overall semiconductor industry.
In his Substack, Zach argues that YC is wrong about LLMs for chip design because they underestimate the difficulty involved in successful chip design. It is difficult for LLMs to simply make up for the talent shortage facing the semiconductor industry. He writes,
And if you’re designing high-value chips in a crowded market, like AI accelerators, performance is one of the major metrics you’re expected to compete on. So it makes sense to spend the additional upfront cost to hire talented RTL designers, build chips with better performance, and deliver more successful products.
Zach concludes by saying that LLMs at best make chip design more economical in certain select scenarios, but most certainly won’t result in LLMs building 100x better chips. Check out the actual article below where he provides more reasoning.
Even if LLMs are not really helpful, the utility of more traditional optimizations for chip design should not be discounted. One useful area where machine-learning algorithms will greatly speed up chip design is in the floorplanning stage. A modern system-on-chip (SoC) has hundreds of irregularly shaped design blocks that must be optimally placed in light of constraints such as minimizing chip area, and not having overlaps. The floorplanning step takes significant amount of human time, effort and experience to get right, sometimes even resulting in suboptimal solutions.
A research team at Intel AI Lab recently demonstrated a machine learning algorithm that they say uses a constraints-aware simulated annealing (CA-SA) approach. They call it parallel simulated annealing with constraints awareness, or Parsac.
The main idea behind it is to start from a random arrangement of blocks and iterate on them evaluating a cost-function every time. The algorithm accepts both good and bad solutions initially in the hope that the bad solutions improve when fixes are implemented to it. As the algorithm iterates, fewer high cost solutions are accepted. The goal behind doing this is to avoid fixating on locally optimal solutions.
Parsac successfully solved commercial-scale floorplanning problems in less than 15 minutes, making it the fastest known floorplanner of its kind. The IEEE Spectrum article on this is an interesting read.
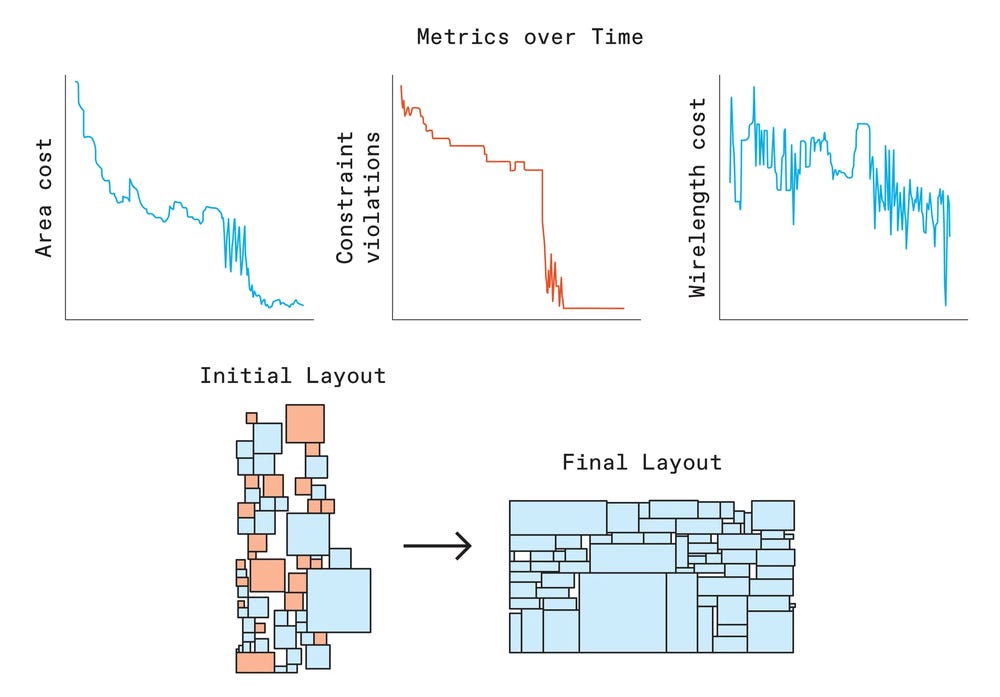
Mapping the Ionosphere with Millions of Android Phones
The region between 50-1500 kilometers above earth is a weakly ionized plasma called the ionosphere. Knowing the condition of the ionosphere is important because GPS systems estimate distance to satellites by measuring the time taken for a radio signal to travel from satellite to receiver. The total electron content (TEC) of the ionosphere affects the speed of radio waves, causing significant errors to geolocation that need to be accounted for using models. Mapping ionosphere TEC is very important to aviation, satellites, navigation and communications.
The delays caused by ionospheric TEC can be used by GPS receivers to actually map it out. Since higher frequency signals have smaller delays through the ionosphere, the discrepancy between travel times of different frequencies can be used to estimate TEC. This has traditionally been done with ground based measurement stations that are expensive to build and maintain.
In a Nature article, researchers have shown that millions of android phones can use their built-in, albeit lower quality (compared to ground based stations built for it) GPS receivers, to create improved ionospheric maps with much improved coverage. Our phones are basically mobile sensors, and there are millions of them in most geographic locations which makes mapping out the ionosphere that much easier instead of building out dedicated stations everywhere. Similarly, the accerelometers in phones have shown to be useful in early detection of earthquakes and phone barometers have been used to improve weather forecasting.
All the data used in ionospheric calculations are open source and come with a python Jupyter notebook that you can use to play with your visualizations. The ionosphere maps over time across the globe is also available as a video (excerpt shown below).
Tools to check symmetry in Analog and RF IC design
Analog and RF design remains a speciality area primarily due to the tacit information required to implement circuits that work well. One such case is correct implementations of symmetry in circuits that have major implications in performance. Differential pairs, and current mirrors in analog, and amplifier cores and switches in RF require careful layout considerations that go beyond just transistor layout techniques such as common centroid.
The routing to the active device, the post layout metal fill, neighboring circuits and even packaging requires symmetry and intentional design to reduce mismatch and provide consistent performance across voltage and temperature. The linked article from the Siemens blog discusses how the requirement of layout symmetry is being addressed using EDA tools.
Correct-by-contruction layout tools attempt to place transistor cells in proper layout orientations, and some open source layout tools such as ALIGN and MAGICAL even attempt to automatically build layouts by detecting symmetries from the netlist level. You can read more about that in my previous article below.
Perfect symmetry is not always possible in layout because of chip area and layout constraints. In such cases, fuzzy symmetry allows for insignificant symmetry mismatches in layouts that won’t significantofly impact circuit performance. Some out of the box tools like Calibre nmPlatform already incorporate some of these advanced symmetry checking features.
If you like this post, please click ❤️ on Substack and subscribe to the publication.
Join our Discord community and get in on the discussion!
The views, thoughts, and opinions expressed in this newsletter are solely mine; they do not reflect the views or positions of my employer or any entities I am affiliated with. The content provided is for informational purposes only and does not constitute professional or investment advice.
Late to this post but enjoyed it a lot. One question though… why wasn’t alphaChip discussed in the section of LLMs for chip design? Sure the approach doesn’t use LLMs but it is using AI to automate stuff so broadly falls in the same category and if it really works as well as it is advertised it’s probably the biggest breakthrough in how chips are designed in the past few years. It would be interesting to have someone not totally onboard the genAI hype train evaluate the merits of alphaChip in a calm and measured way.
+1 vote for this format becoming a regular fixture in VNL